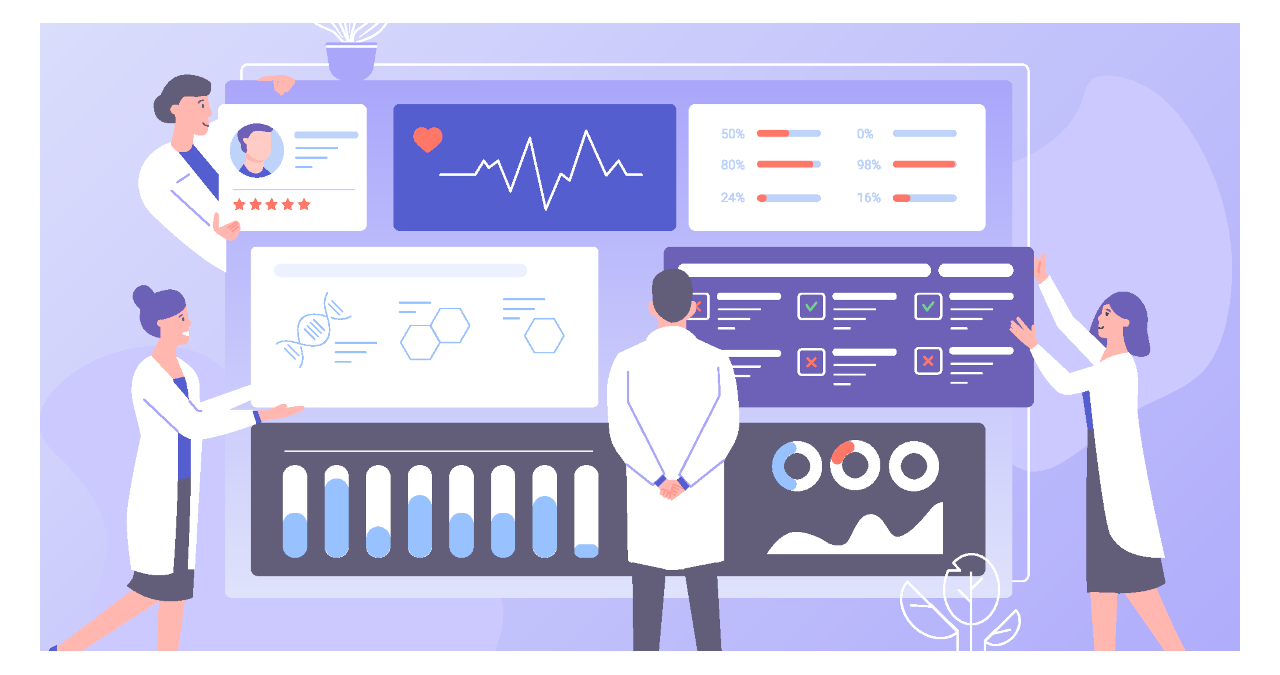
Editor’s Note: This content has been excerpted from the article “Using Data Analytics to Improve Hospital Quality Performance,” which was published in the Journal of Healthcare Management, vol. 65, no. 4. It has been edited down for length. The full article received the 2022 Edgar C. Hayhow Award and the authors will be recognized during the Congress on Healthcare Leadership.
The quality-cost trade-off is a fundamental challenge for healthcare providers. While cost is readily quantifiable, the measurement of quality is considerably more challenging. Quality is sometimes defined in terms of structure, process and outcomes. However, most stakeholders, including patients, insurers and policymakers, are often unaware of a hospital’s structure and processes, and therefore hospital quality is often measured primarily in terms of patient outcomes.
The Challenge of Trying to Quantify Complex Care Measures
Among the many outcome measures used for hospitals, perhaps the most common are mortality rate, average length of stay and readmission rate. A successful hospital visit is one in which the patient is discharged alive after a reasonable period and does not need to return. These measures are often adjusted to reflect the case and patient characteristics of the hospital relative to those at other hospitals. Typically, these measures are analyzed separately. Moreover, these analyses often do not provide hospital management with specific targets: How low should the hospital’s mortality rate, readmission rate and length of stay be, given its patient and case characteristics?
The measurement of hospital quality has been a challenge for decades. The complexity of a hospital’s services implies that the measurement of its quality is certain to be similarly complex. Every hospital provides a broad range of medical and surgical services to inpatients and outpatients. Highly trained professional staff must provide these services by using advanced technology and skills. In addition, patients present with various mixes of diagnoses and differing pre-existing patient and case characteristics.
Analysts use a plethora of measures, with each measure designed to address one specific aspect of quality. Some measures pertain only to a certain subpopulation of patients, such as surgical or obstetric, and are useful for measurement of quality within that subset. Others, such as average length of stay, mortality rate and readmission rate, are applied more generally. These are useful in examining overall hospital performance. These measures require adjustment for patient and case characteristics and are often measured in isolation. The purpose of this study was to present a methodology for integrating multiple outcome measures into a comprehensive model appropriate for guiding hospital managers and healthcare decision-makers in their quest to improve quality.
Creating a New Model to Measure Hospital Performance
The objective of our study was to build a unified quality performance model for hospitals using publicly available data. We obtained data from the New York State Department of Health’s Statewide Planning and Research Cooperative System database for our model, which had three outcome measures that we wished to make smaller (deaths, readmissions, average length of stay). Because this was a performance model rather than an economic efficiency model, we excluded costs, which are affected significantly by local economic conditions.
The model incorporates risk adjustment by recognizing that different hospitals treat patients with different patient and case characteristics that may affect one or more of the outcome measures. The model is flexible in the sense that any combination of outcome measures and patient and case characteristics may be used if there are data available for these measures for each hospital in the defined set of hospitals.
Using our new model, we included four site characteristics. With our data envelopment analysis model structure, we used logistic regression to analyze the output. We extracted data for 2,233,214 discharges in 2014 from 183 hospitals in New York State. We found that 20.8% of the facilities were on the quality performance frontier—20.6% of the not-for-profit facilities and 21.4% of the other facilities. Hospitals with more discharges performed better with respect to mortality, readmission and average length of stay. We found no difference in performance between not-for-profit hospitals and others.
Study Results and Takeaways
We concluded that 79.2% of hospitals could improve their quality of care. As an upper bound, if all hospitals increased each quality factor performance to 100%, there would have been 11,722 (24.8%) fewer deaths, 17,840 (15.8%) fewer readmissions and the statewide average length of stay would have been 0.71 days (13.5%) less.
Our approach is not to deny the importance of structure and process in overall quality, but rather to focus on outcomes and help hospital administrators seeking to improve organizational performance. We believe that this model can also assist the New York State Department of Health as it monitors statewide hospital performance. Repeating this analysis annually will lead to improved hospital quality performance and better healthcare. Moreover, the model uses data that are already readily publicly available in the New York State Department of Health database. The model can also be performed over a multiyear period to uncover trends in quality performance.
Read more about how our team used data to build a quality performance model for hospitals.
Christine Pitocco, PhD, is research professor, Stony Brook (N.Y.) University, and can be reached at Christine.Pitocco@StonyBrook.edu.